Dando un salto cuántico, la inteligencia artificial (IA) es una tecnología clave para la industria automotriz
Dando un salto cuántico, la inteligencia artificial (IA) es una tecnología clave para la industria automotriz
Cada vez más funciones de los vehículos se basan en la inteligencia artificial. Sin embargo, los procesadores convencionales e incluso los chips gráficos están llegando cada vez más a sus límites en lo que respecta a los cálculos necesarios para las redes neuronales. Porsche Engineering informa sobre nuevas tecnologías que acelerarán los cálculos de IA en el futuro.
La inteligencia artificial (IA) es una tecnología clave para la industria automotriz, y el hardware rápido es igualmente importante para los complejos cálculos de back-end involucrados. Después de todo, en el futuro solo será posible llevar nuevas funciones a la producción en serie con computadoras de alto rendimiento. “La conducción autónoma es una de las aplicaciones de IA más exigentes de todas”, explica el Dr. Joachim Schaper, Gerente Senior de IA y Big Data en Porsche Engineering. “Los algoritmos aprenden de una multitud de ejemplos recopilados por vehículos de prueba que utilizan cámaras, radares u otros sensores en el tráfico real”.

dr. Joachim Schaper, Gerente Senior de IA y Big Data en Porsche Engineer
Los centros de datos convencionales son cada vez más incapaces de hacer frente a las crecientes demandas. “Ahora lleva días entrenar una sola variante de una red neuronal”, explica Schaper. Entonces, en su opinión, una cosa está clara: los fabricantes de automóviles necesitan nuevas tecnologías para los cálculos de IA que puedan ayudar a que los algoritmos aprendan mucho más rápido. Para lograr esto, se deben ejecutar en paralelo tantas multiplicaciones de matriz vectorial como sea posible en las complejas redes neuronales profundas (DNN), una tarea en la que se especializan las unidades de procesamiento de gráficos (GPU). Sin ellos, los increíbles avances en IA de los últimos años no habrían sido posibles.
50 veces el tamaño de una GPU
Sin embargo, las tarjetas gráficas no se diseñaron originalmente para el uso de IA, sino para procesar datos de imagen de la manera más eficiente posible. Están cada vez más al límite cuando se trata de algoritmos de entrenamiento para la conducción autónoma. Por lo tanto, se requiere hardware especializado en IA para cálculos aún más rápidos. La empresa californiana Cerebras ha presentado una posible solución. Su Wafer Scale Engine (WSE) se adapta de manera óptima a los requisitos de las redes neuronales al combinar la mayor potencia informática posible en un chip de computadora gigante. Es más de 50 veces el tamaño de un procesador de gráficos normal y ofrece espacio para 850 000 núcleos informáticos, más de 100 veces más que en una GPU superior actual.
Además, los ingenieros de Cerebras han conectado en red los núcleos computacionales junto con líneas de datos de gran ancho de banda. Según el fabricante, la red del Wafer Scale Engine transporta 220 petabits por segundo. Cerebras también ha ampliado el cuello de botella dentro de las GPU: los datos viajan entre la memoria y la unidad de cómputo casi 10 000 veces más rápido que en las GPU de alto rendimiento, a 20 petabytes por segundo.

Chip gigante: el Wafer Scale Engine de Cerebras combina una enorme potencia informática en un solo circuito integrado con una longitud lateral de más de 20 centímetros.
Para ahorrar aún más tiempo, Cerebras imita un truco del cerebro. Allí, las neuronas funcionan solo cuando reciben señales de otras neuronas. Las muchas conexiones que están actualmente inactivas no necesitan ningún recurso. En las DNN, por otro lado, la multiplicación de matriz vectorial a menudo implica multiplicar por el número cero. Esto cuesta tiempo innecesariamente. Por lo tanto, Wafer Scale Engine se abstiene de hacerlo. “Todos los ceros se filtran”, escribe Cerebras en su libro blanco sobre el WSE. Entonces, el chip solo realiza operaciones que producen un resultado distinto de cero.
Un inconveniente del chip es su alto requerimiento de energía eléctrica de 23 kW y requiere refrigeración por agua. Por lo tanto, Cerebras ha desarrollado su propia carcasa de servidor para su uso en centros de datos. El Wafer Scale Engine ya se está probando en los centros de datos de algunos institutos de investigación. El experto en inteligencia artificial Joachim Schaper cree que el chip gigante de California también podría acelerar el desarrollo automotriz. “Al usar este chip, el entrenamiento de una semana podría reducirse teóricamente a unas pocas horas”, estima. “Sin embargo, la tecnología aún tiene que demostrarlo en pruebas prácticas”.
Luz en lugar de electrones
A pesar de lo inusual que es el nuevo chip, al igual que sus predecesores convencionales, también funciona con transistores convencionales. Empresas como Lightelligence y Lightmatter, con sede en Boston, quieren utilizar el medio de la luz mucho más rápido para los cálculos de IA en lugar de la electrónica comparativamente lenta, y están construyendo chips ópticos para hacerlo. Por lo tanto, los DNN podrían funcionar “al menos varios cientos de veces más rápido que los electrónicos”, escriben los desarrolladores de Lightelligence.
“Con Wafer Scale Engine, una semana de entrenamiento teóricamente podría reducirse a solo unas pocas horas”. Dr. Joachim Schaper, gerente sénior de IA y Big Data en Porsche Engineering
Para ello, Lightelligence y Lightmatter utilizan el fenómeno de la interferencia. Cuando las ondas de luz se amplifican o anulan entre sí, forman un patrón claro-oscuro. Si dirige la interferencia de cierta manera, el nuevo patrón corresponde a la multiplicación vector-matriz del patrón anterior. Entonces, las ondas de luz pueden “hacer matemáticas”. Para que esto sea práctico, los desarrolladores de Boston grabaron diminutas guías de luz en un chip de silicio. Como en un tejido textil, se cruzan varias veces. La interferencia tiene lugar en los cruces. En el medio, diminutos elementos calefactores regulan el índice de refracción de la guía de luz, lo que permite que las ondas de luz se desplacen entre sí. Esto permite controlar su interferencia y realizar multiplicaciones vector-matriz.
Sin embargo, las empresas de Boston no prescinden por completo de la electrónica. Combinan sus computadoras livianas con componentes electrónicos convencionales que almacenan datos y realizan todos los cálculos, excepto las multiplicaciones de vectores y matrices. Estos incluyen, por ejemplo, las funciones de activación no lineal que modifican los valores de salida de cada neurona antes de pasar a la siguiente capa.

Computación con luz: el chip Envise de Lightmatter utiliza fotones en lugar de electrones para calcular redes neuronales. Los datos de entrada y salida son suministrados y recibidos por electrónica convencional.
Con la combinación de computación óptica y digital, los DNN se pueden calcular extremadamente rápido. “Su principal ventaja es la baja latencia”, explica Lindsey Hunt, portavoz de Lightelligence. Por ejemplo, esto permite que la DNN detecte objetos en imágenes más rápido, como peatones y usuarios de scooters eléctricos. En la conducción autónoma, esto podría dar lugar a reacciones más rápidas en situaciones críticas. “Además, el sistema óptico toma más decisiones por vatio de energía eléctrica”, dijo Hunt. Eso es especialmente importante ya que el aumento de la potencia informática en los vehículos se produce cada vez más a expensas de la economía de combustible y la autonomía.
Las soluciones de Lightmatter y Lightelligence se pueden insertar como módulos en computadoras convencionales para acelerar los cálculos de IA, al igual que las tarjetas gráficas. En principio, también podrían integrarse en vehículos, por ejemplo, para implementar funciones de conducción autónoma. “Nuestra tecnología es muy adecuada para servir como motor de inferencia para un automóvil autónomo”, explica Lindsey Hunt. El experto en inteligencia artificial Schaper tiene una opinión similar: “Si Lightelligence tiene éxito en la construcción de componentes adecuados para automóviles, esto podría acelerar en gran medida la introducción de funciones complejas de inteligencia artificial en los vehículos”. La tecnología ya está lista para el mercado: la compañía está planeando sus primeras pruebas piloto con clientes en el año 2022.
La computadora cuántica como un turbo AI
Las computadoras cuánticas están algo más alejadas de la aplicación práctica. Ellos también acelerarán los cálculos de IA porque pueden procesar grandes cantidades de datos en paralelo. Para ello, trabajan con los llamados “qubits”. A diferencia de la unidad de información clásica, el bit, un qubit puede representar los dos valores binarios 0 y 1 simultáneamente. Los dos números coexisten en un estado de superposición que solo es posible en la mecánica cuántica.
“Cuanto más complicados son los patrones, más dificultad tienen las computadoras convencionales para distinguir clases”. Heike Riel, directora de IBM Research Quantum Europa/África
Las computadoras cuánticas podrían impulsar la inteligencia artificial cuando se trata de clasificar cosas, por ejemplo, en el tráfico. Hay muchas categorías diferentes de objetos allí, incluidas bicicletas, automóviles, peatones, señales, carreteras secas y mojadas. Difieren en términos de muchas propiedades, razón por la cual los expertos hablan de “reconocimiento de patrones en espacios de dimensiones superiores”.
“Cuanto más complicados son los patrones, más difícil es para las computadoras convencionales distinguir las clases”, explica Heike Riel, quien dirige la investigación cuántica de IBM en Europa y África. Eso se debe a que con cada dimensión, se vuelve más costoso calcular la similitud de dos objetos: ¿Qué tan similares son un conductor de e-scooter y un usuario de andador tratando de cruzar la calle? Las computadoras cuánticas pueden funcionar de manera eficiente en espacios de alta dimensión en comparación con las computadoras convencionales. Para ciertos problemas, esta propiedad podría ser útil y dar como resultado que algunos problemas se resuelvan más rápido con la ayuda de las computadoras cuánticas que con las computadoras convencionales de alto rendimiento.
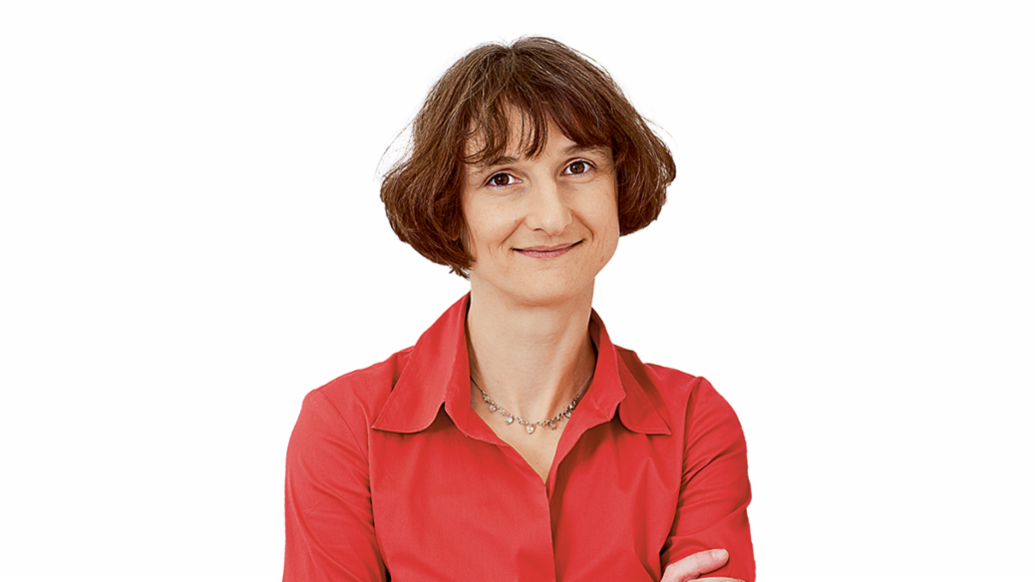
Heike Riel, directora de IBM Research Quantum Europa/África
Los investigadores de IBM han analizado modelos estadísticos que se pueden entrenar para la clasificación de datos. Los resultados iniciales sugieren que los modelos cuánticos inteligentemente elegidos funcionan mejor que los métodos convencionales para ciertos conjuntos de datos. Los modelos cuánticos son más fáciles de entrenar y parecen tener una mayor capacidad, lo que les permite aprender relaciones más complicadas.
Riel admite que, si bien las computadoras cuánticas actuales se pueden usar para probar estos algoritmos, aún no tienen una ventaja sobre las computadoras convencionales. Sin embargo, el desarrollo de las computadoras cuánticas avanza rápidamente. Tanto el número de qubits como su calidad aumentan constantemente. Otro factor importante es la velocidad, medida en operaciones de capa de circuito por segundo (CLOPS). Este número indica cuántos circuitos cuánticos pueden ejecutarse en la computadora cuántica por vez. Es uno de los tres criterios de rendimiento importantes de una computadora cuántica: escalabilidad, calidad y velocidad.
En un futuro previsible, debería ser posible demostrar la superioridad de las computadoras cuánticas para ciertas aplicaciones, es decir, que resuelven problemas de manera más rápida, eficiente y precisa que una computadora convencional. Pero la construcción de una computadora cuántica potente, con errores corregidos y de propósito general aún llevará algún tiempo. Los expertos estiman que llevará al menos otros diez años. Pero la espera podría valer la pena. Al igual que los chips ópticos o las nuevas arquitecturas para computadoras electrónicas, las computadoras cuánticas podrían ser la clave de la movilidad del futuro.
En breve
Cuando se trata de cálculos de IA, no solo los microprocesadores convencionales, sino también los chips gráficos, ahora están llegando a sus límites. Por lo tanto, empresas e investigadores de todo el mundo están trabajando en nuevas soluciones. Los chips en formato oblea y los ordenadores ligeros están cerca de hacerse realidad. En unos años, estos podrían complementarse con computadoras cuánticas para cálculos particularmente exigentes.
1
COMPARTE
|
Leave a Reply
Want to join the discussion?Feel free to contribute!